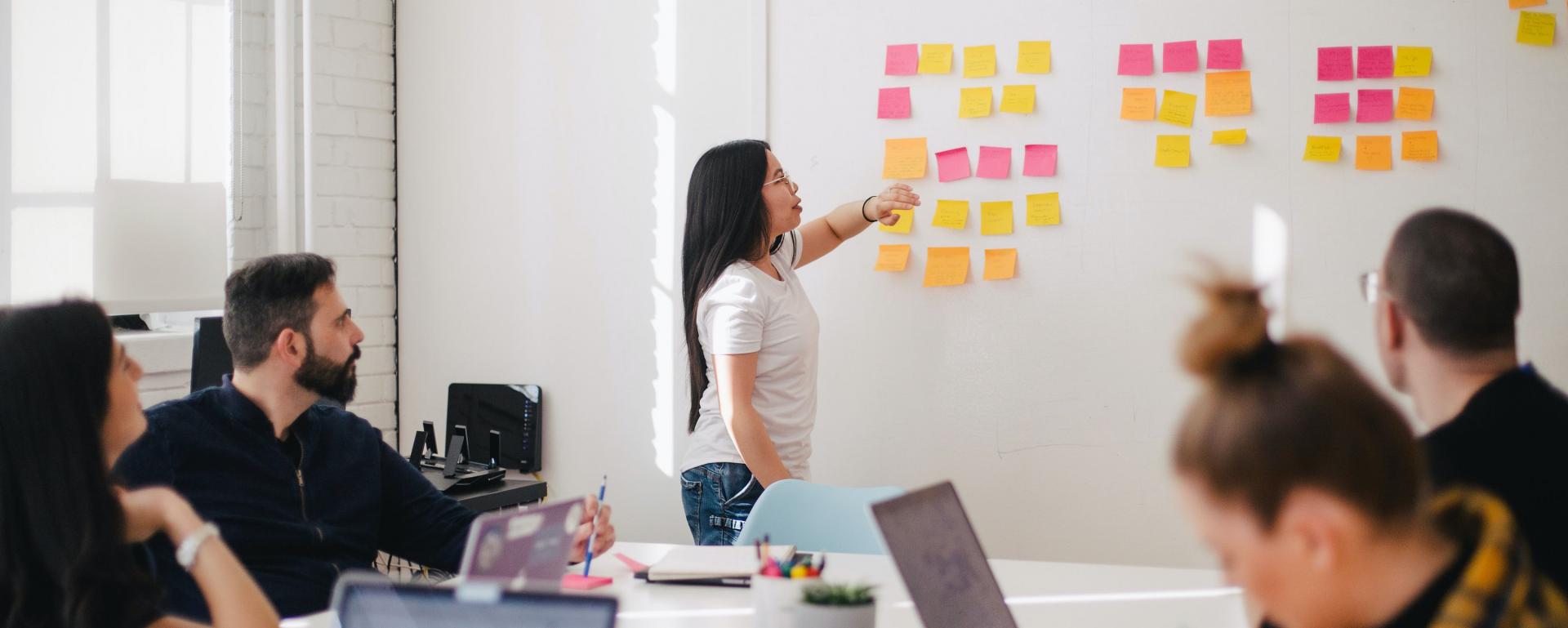
Wouldn’t it be exciting to gather evidence about the impact of coaching services to support startups? It was with this idea that we applied for an Innovation Growth Lab grant back in 2015. Driven by our delivery partner, an accelerator with a lot of experience offering business coaching services to tech startups, we set out to evaluate the impacts of their coaching. We decided that a randomised controlled trial would be an appropriate but innovative method and were successful in our application to the IGL Grants Programme. A few years on, here are our reflections and lessons that we hope will be useful to others undertaking similar experiments.
Plan
The plan was clear – a two-armed RCT testing the effect of business coaching on venture survival, carried out in the German region ‘Baden-Württemberg’, one of the most innovative regions in the EU. Among the population of tech startups, we had planned to sample 450over three years as part of the experiment. The business coaching intervention was to be carried out by our delivery partner to 150 of these young ventures. Funded by budgets of the European Social Fund, the coaching could only be provided to 50 startups per year. We calculated the statistical power and potential effect sizes for the later data analysis, elaborated on a proxy to measure survivability stages of the sampled startups, planned how to survey them and collect follow-up data, prepared and tested the randomisation process. We were confident that nothing fundamental could go wrong… however, the reality proved different!
Reality
After running the RCT for two years, we started analysing the data collected in the first half of the experiment. Partly lifting the anonymisation of the data to match startups with the received coaching hours, it turned out that attrition between groups by far exceeded our expectation. And even worse: we had 64 startups that had received coaching, even through they were not part of the sample. Of course, we could still analyse the data, but the findings were rather unspectacular and even slightly counterintuitive. Comparing the survival capability between the contaminated treatment control groups, data did not show any statistically significant difference. Yet, when disrespecting the ITT (intention to treat) principle, the group which had actually been treated performed better than the randomised treatment group. We have come up with two theories that could explain this difference: either business coaching in fact contributed to an increase in survivability and we would just need more startups and a longer experiment duration to find proof for the effect, or we might have witnessed some sort of self-selection bias where those startups that have better survival prospects are also more prone to request receiving business coaching support.
What went wrong
-
The sample size was smaller than expected, which we discovered early. To increase the number of startups attending training events (which was our experiment entry point), our delivery partner appointed an additional employee to push advertising of the compact training events.
-
The response rate of our follow-up data collection that measured the main outcome via a questionnaire was low. Therefore, we carried out personal phone calls to every participant, organised a prize draw among those who replied, and conceptualised and offered a ‘venture health check’ by providing a comparison with other comparable startups. All efforts eventually increased the response rate from 30% to 57%.
-
The attrition between groups was very high. Due to non transparent data management and a lack of communication, we noticed this only after half of the experiment had been conducted. According to our delivery partner, more effort was placed on convincing those supposed to receive the intervention to actually take part in it.
-
Unexpectedly, we had a large number of ’external teams‘ as a significantly confounding factor. Unfortunately, we could not avoid this, since the state-funded intervention was theoretically free to everyone requesting to receive it, until the budget was fully used.
-
Finally, the state unexpectedly cancelled the delivery partner’s budget for the interventions in 2018. Well, there was nothing we could do about that. All of a sudden, our experiment was finished.
What we learned
-
Expect the unexpected
- plan carefully, realistically and reliably
- past experience may not hold in the future: thus, avoid optimism, think of measures to increase sample size (incentives may be difficult in the business context!)
-
Agree on duties
- precisely define roles and responsibilities with delivery partners
- have a written document including milestones, processes, objectives, and communication terms to detect deviations from the plan early
-
Strive for data
- if possible, avoid relying on questionnaire data to eliminate self-selection bias and the risk of not obtaining the outcome data needed
- if you rely on questionnaire data to measure the outcome, make sure that this data will be provided by the experiment participants; think about ways to ensure participants provide necessary follow-up data, even if they are unaware that they are taking part in an experiment
-
Detect invaders
- attrition and contamination are real threats that can creep in wherever there are weaknesses
- avoid interventions that can be freely accessed by people other than the trial participants (open support programmes)
-
Keep control
- interventions funded by state programmes can be tricky as there may be limitations imposed which could make a proper randomisation hard to conduct
- ensure that a termination of funding is not possible
-
Stay pragmatic
- ’trickle samples‘ require considerable coordination efforts
- very careful planning of processes is recommended to ensure an accurate data collection over time and an adequate execution of the randomisation
- where possible, apply straightforward, pragmatic approaches over ones which are analytically more complicated
So now you know about our story and about what potentially can go wrong with your RCT… If you have any questions or comments, leave us a message and we are happy to get in contact and provide further advice to researchers conducting RCTs in a similar context!