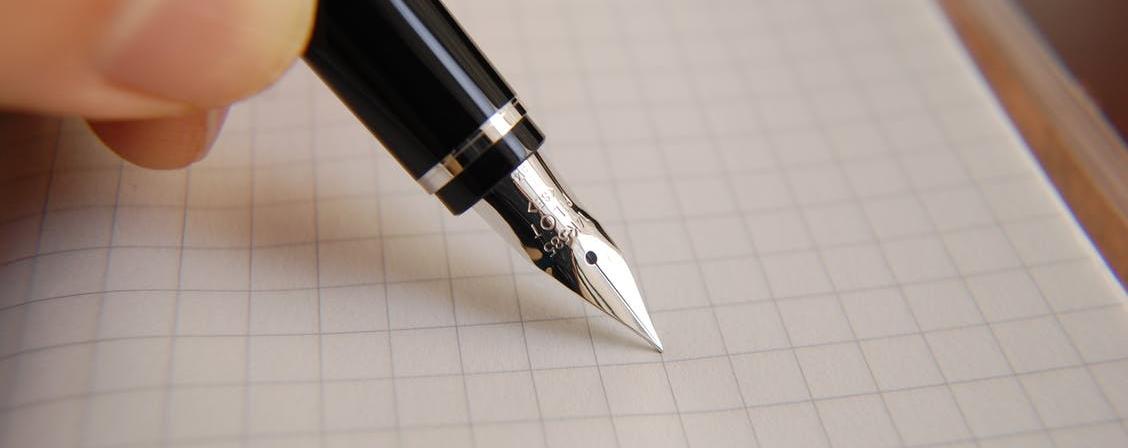
At IGL2017 we will be launching the first version of our online toolkit, which we hope will help those delivering trials in the area of innovation, entrepreneurship and business growth policy - complementing the IGL guide published last year.
To develop the toolkit we have been pondering all the ways that randomised controlled trials (RCTs, or simply trials) can be used to inform policy and the elements that need to be planned for trials to be used successfully. We have also had the benefit of conversations with several researchers, practitioners and policymakers to learn from their experience of delivering trials.
In this blog we put forward seven tips for those looking at when and how trials could be used.
1) Be on the look-out for opportunities
We remain a long way from policy experimentation being the central feature of policy development that IGL believes it should be. Introducing trials may therefore require starting with small rapid experiments or seizing opportunities as they arise.
The opportunity can arise when programmes are over subscribed with suitable applicants - as was the case with this trial of innovation vouchers. A lottery in these circumstances may be readily accepted as the most fair and cost effective way to allocate support. But when you are relying on over subscription to generate the trial, it is worth planning for how to respond if later there is pressure to boost numbers in the programme.
Another situation that presents an opening for a trial may come when a programme needs changing but there is no agreement over how to do so. Perhaps cost savings are demanded, with some calling to preserve the programme design but reduce the numbers supported whilst others favour a switch to lower-cost alternatives (e.g. one-to-many advice rather than one-to-one). The inclusion of a trial that pilots these alternatives could resolve the matter by providing evidence that is sufficiently robust to change the minds of those on either side of the argument.
2) Set out the theory of change for the programme or intervention
When designing a trial we suggest using a logic model to set out the different elements involved in the delivery of the programme and how these connect to the desired outcomes. This logic model will have most value when you have gone into the ‘theory of change’ behind these connections. This requires you to consider in detail the rationale for the programme (or intervention), what needs to be delivered and then the ‘cause and effect’ assumptions that must hold for the ultimate objectives to be realised.
This framework can be very useful when assessing how suitable a programme is for the inclusion of a trial; the participants that you will want to recruit; the data collection measures that will need to be in place and the time period over which results will become available. As we will discuss in the forthcoming toolkit, it can also be a great way to generate ideas of where a trial can be used to inform and improve programme delivery.
3) A trial will work best when you are able to set out clear outcomes objectives
For impact evaluations a trial will be most suitable when your policy objectives for it can be captured within a small number of SMART targets [1]. Trials will be difficult if the impacts could occur across a large and disparate number of outcomes, especially if there is no certainty on the importance or likely timing of changes - i.e. you won’t know what success looks like even when you see it.
If a trial isn’t suitable for an impact evaluation of the entire programme, the logic model could identify where trials might prove useful. Perhaps the scope of the trial can be narrowed to determine the causal impacts of the programme on more immediate outcomes variables - e.g. testing whether school-based entrepreneurial education increases capabilities and intentions, but not business startups.
4) Test key ‘cause and effect’ assumptions before launching the trial
We recommend you challenge the assumptions set out in your theory of change. For example, does the linear framework of the logic model mean you are only presenting one of many scenarios of how the intervention could meet your final objectives?
Programmes do not always get used and deliver results in the way that was first envisaged. For example, a logic model for an innovation vouchers programme could specify that impacts will come from participant businesses collaborating for the first time with external researchers. However, in practice the impact may instead come from deepening levels of collaboration, which would be missed if trial outcome measures only captured new connections.
If you are unsure, it can be beneficial to test assumptions in advance of the trial, perhaps by running a small pilot.
5) Find the optimum place to implement randomisation
Where and how randomisation is implemented within a programme can have a huge impact on the power of a trial - i.e. the level of change in outcomes that you can be confident of detecting and thereby the trial’s ability to answer the research question.
To illustrate, consider a simple trial for a grant programme. The trial has been designed such that after eligibility checks participants are randomly allocated to either receive the grant or to a control that does not. However, eligibility checks are expensive and so later the decision is taken to conduct these after the point of randomisation.
Suppose only half of the applicants in the treatment group pass the eligibility check. Whilst the minimum detectable effect size of the trial is the same, this impact now has to be generated by half the sample [2] - so the impacts on those actually using the grant now has to be twice as large to be detected.
6) Look at other trials and evaluations of the same type of programme
If your main interest is in expanding the knowledge frontier on ‘what works’ then you may not want to proceed with a trial if a similar trial has already been conducted. But even if your primary interest is the impact of your specific programme in its context we would recommend looking for other trials. For example, you may learn from their experience with recruiting participants into the trial, designing survey questions to measure outcomes or the potential scale and nature of impacts you could expect.
7) Keep a record of the key information about your trial and register details before delivery starts
Whether you are a researcher or policymaker, a trial protocol should be used to set out the key feature of your research plan and we also strongly recommend you then register the trial - eg in the AEA registry and also our own IGL database.
As discussed in the IGL guide this enhances the credibility of your research by removing any concerns that the programme ‘narrative’ is set by the few outcome measures for which positive impacts were found. It also helps reduce publication bias (important to learn from what doesn’t as well as what does work) and helps to promote collaboration and avoid duplication. Making the micro data available can also help to maximise the externality benefits of a trial, and gives your results more credibility.
A trial protocol can also be a vital tool for knowledge management, especially for long-term trials and those that might be exposed to changes in the policy landscape - i.e. those involved in commissioning and implementing the trial are not around when it comes to extracting the findings.