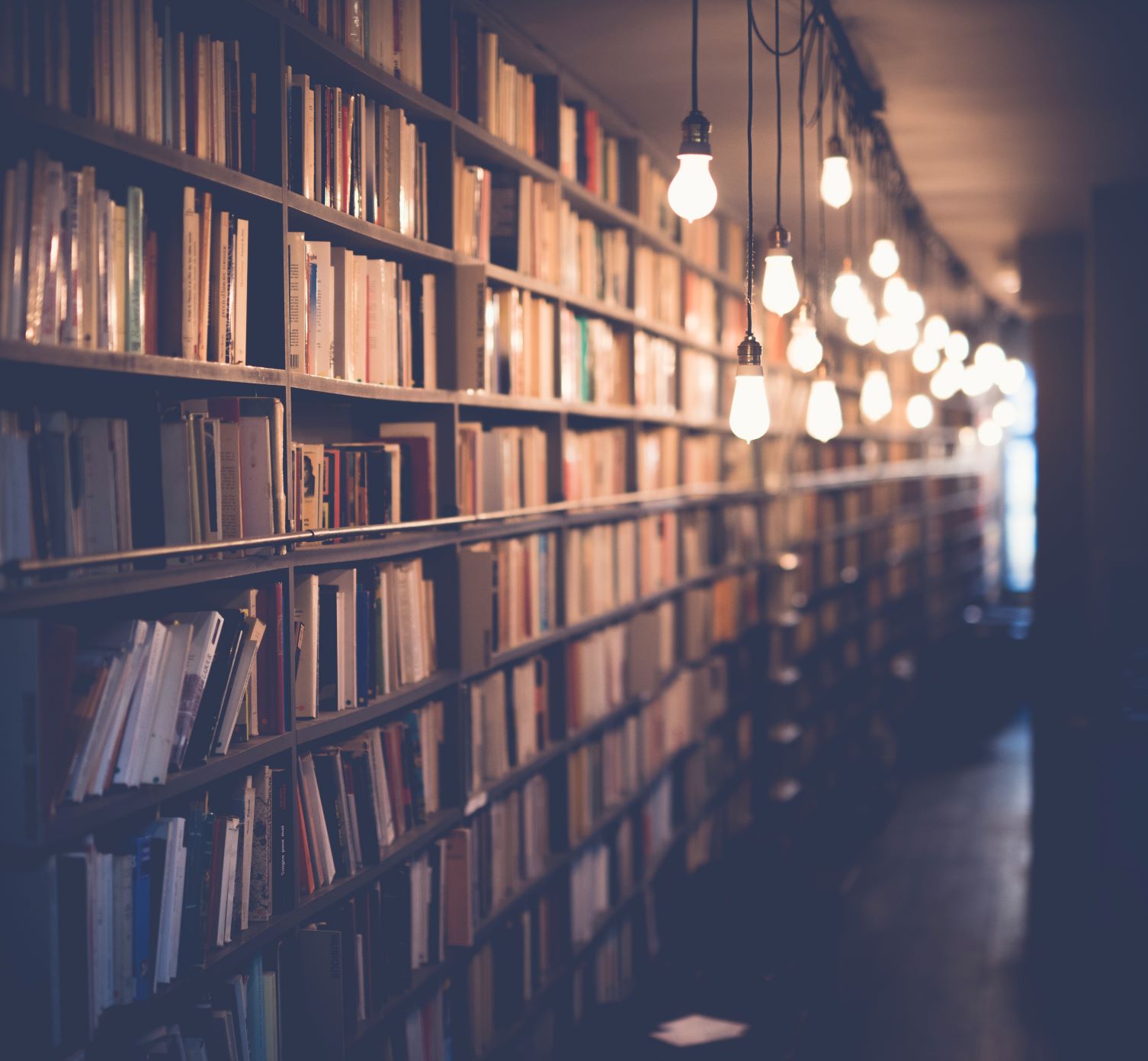
Blog
How experimental learning takes place
24 March 2023
Experimental approaches to innovation policy have the potential to vastly improve how we support scientific and technological discovery, entrepreneurship and other high growth sectors within our economies. As the role of innovation agencies shifts to meet changing demands, be they mission-oriented and focused on big societal challenges or responsive to the exponential advancement of technology, becoming experimental as a policymaker has become far more pertinent. As the Policy Learning Designer for the Innovation Growth Lab (IGL), my role is to support IGL’s partners and the wider policy community to share and learn from new policy ideas. In close collaboration with IGL’s experimental researchers, my practice as a designer carefully lays the groundwork before experimental practice takes place. Over the past year, I have reflected on how myself and others view experimental learning taking place.
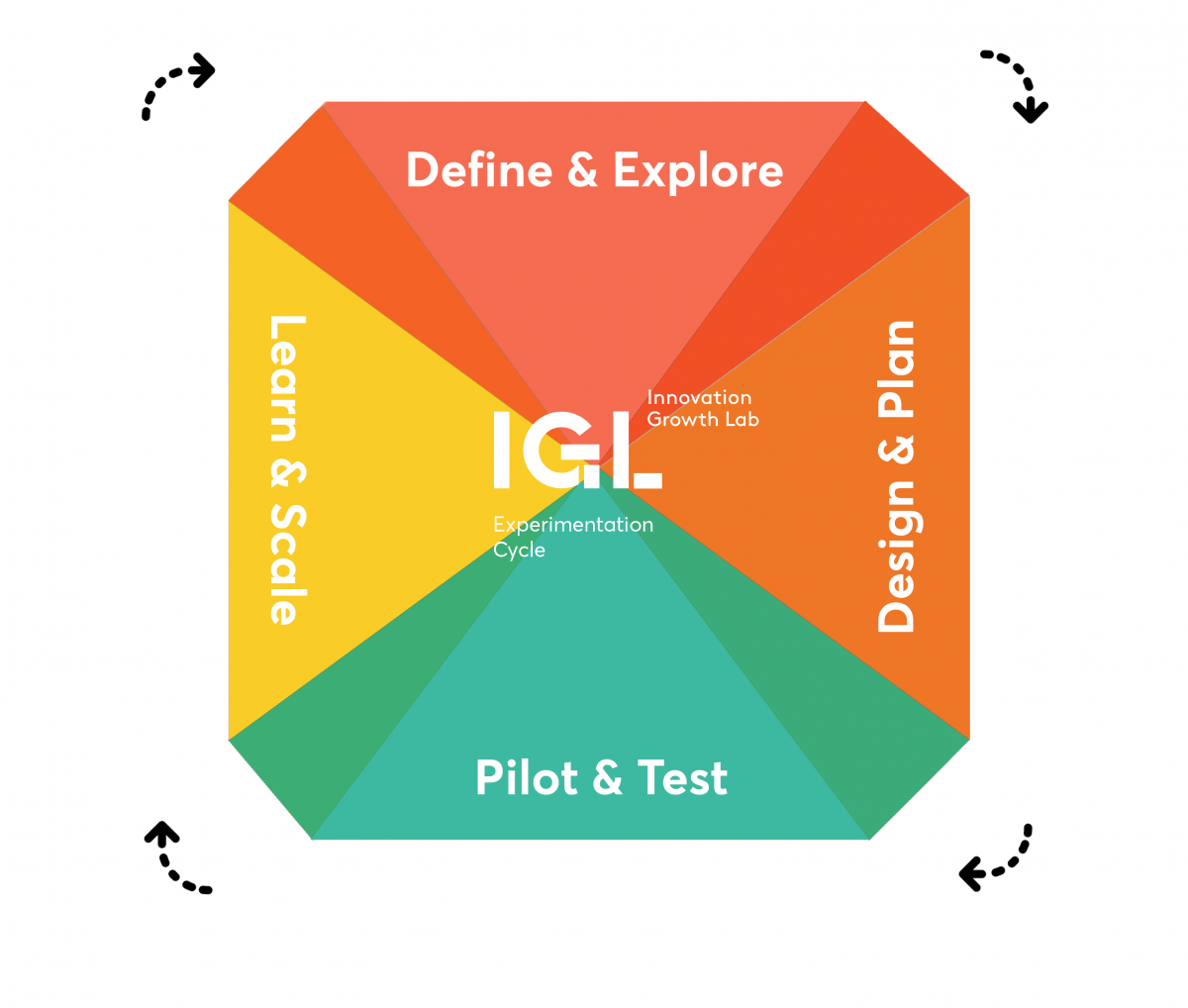
The four phases of IGL’s virtuous experimental cycle
Experimental learning must be experiential
How experimentation is applied to policymaking cannot be taught through a textbook, most of what you learn will need to be experienced to be fully understood and conscientiously adopted (later becoming an unconscious habit). Nesta has previously produced a number of resources on how a policymaker could develop the skills, behaviours and attitudes required for experimentation and true problem solving to take place in public service. At IGL, we deploy a number of tools and resources to support experimental journeys that begin with correctly diagnosing policy problems and end with structured learning on how to run individual experiments (ranging from small pilots to randomised controlled trials).
However, none of these tools replace practical lessons that come from learners trying out what they have learnt in their day-to-day roles, and real infrastructures and processes that need to be in place. Often it is through experiential learning that the first barriers a learner has to confront are met. On the one hand, this can lead to the realisation that experimentation requires strong resilience, imagination and agility. But beyond mindset, in order for experiments to be designed and run successfully, organisations need to have the capabilities and structures in place to support such activity. For example, having good data infrastructures or legal buy-in is crucial.
Rarely will the first experiment idea be the crux that enables experiments to take place as originally imagined. Far more often, the first idea or experiment pursued is an exemplar case for how much of the learner’s understanding will need to be translated to their context (which will often also mean a degree of change management at an organisational level) and reconceptualised for experimentation to take place.
IGL’s recently designed learning programme for development agencies split the syllabus in two, first focusing on helping agencies to lay the foundation for experimentation to take place by exploring opportunities within existing contexts. Later, the syllabus moved on to designing and running effective randomised controlled trials (RCTs) for those same contexts. Not all hypotheses we wish to test are suitable for an RCT. However, the principles to design an RCT ensure a robust framework is in place for other pilots or quasi-experiments to take place. This sets the reasoning behind why at IGL we use RCTs as the benchmark for other experiments which do not look to prove causal impact but lead to robust evidence being generated nonetheless.
The virtuous experimental cycle unlocks a series of steps to achieve desired outcomes
Working with the evidence you have
As part of clarifying the learning journey IGL wishes to take its partners and collaborators through, my colleagues and I have also worked to define the ‘virtuous experimentation cycle’. This process map is virtuous in that it is how we would like the design and running of randomised controlled trials to take place – as opposed how we expect it to actually happen. The steps, which are still a work in progress and open to feedback, mark pivotal points in the process to design and run an RCT well – i.e. the steps up to and around those covered in our guide to the actual running of an RCT.
We know that in practice both experienced and novice experimenters will likely not follow the cycle in a linear fashion – the reason why learning is wedged firmly across all phases. For example, when we review existing evidence we may be working with robust data sets indicating past performance of a programme. In a perfect world, this data could help us to design and run a shadow experiment that tests the immediate outcome of a tweak to the programme design by applying the new process to historic data – e.g. applying a new assessment process to historic applications. However, we might find in the “real world” the existing evidence is more anecdotal than quantitative. In such a case, we wouldn’t suggest we drop the original experiment idea and go back to the drawing board here. Instead we would encourage agility, for example by applying tools for data analysis we can extract more data from what has already been collected. The aim is always to work with what is available and move forward towards action.
Beyond a few champions and towards cultures of change
The biggest reflection in recent years has been the importance of weaving our capacity building work with experimentation champions across whole organisations, to ensure our work is attempting to shift cultures as well as individual practice. Those who design and run experiments are often not synonymous with those who are in a position to use the evidence to inform policy decisions. Looking at the bigger picture of how organisations function means considering how change takes place – this requires the involvement of decision makers at pivotal moments in the experimental process. For example, when reviewing evidence this is a great moment to get all relevant stakeholders to question existing assumptions and collectively choose which focal areas to run an experiment, ensuring buy-in from the start. It is also necessary to discuss the resources that will be required to design and run the experiment, which may extend beyond the usual suspects. For example, it is often necessary to involve the legal department of an organisation early or if the data team sits separately from the programme development team, these units would also need to be brought together from the start.
Final remarks
Getting innovation policy to embrace experimentation in theory and in practice is as much about building capacity as it is about shifting whole systems. There is no linear approach or model that can be replicated but through our work at IGL we know there are common lessons that can support the experimental learners we work with. The principles, processes, tools and resources we share set a helpful framework to discuss what experimentation looks like in each individual context. However, it can never replace the expertise of those delivering this work who must embark on experiential learning to translate theory into their individual practices and environments.