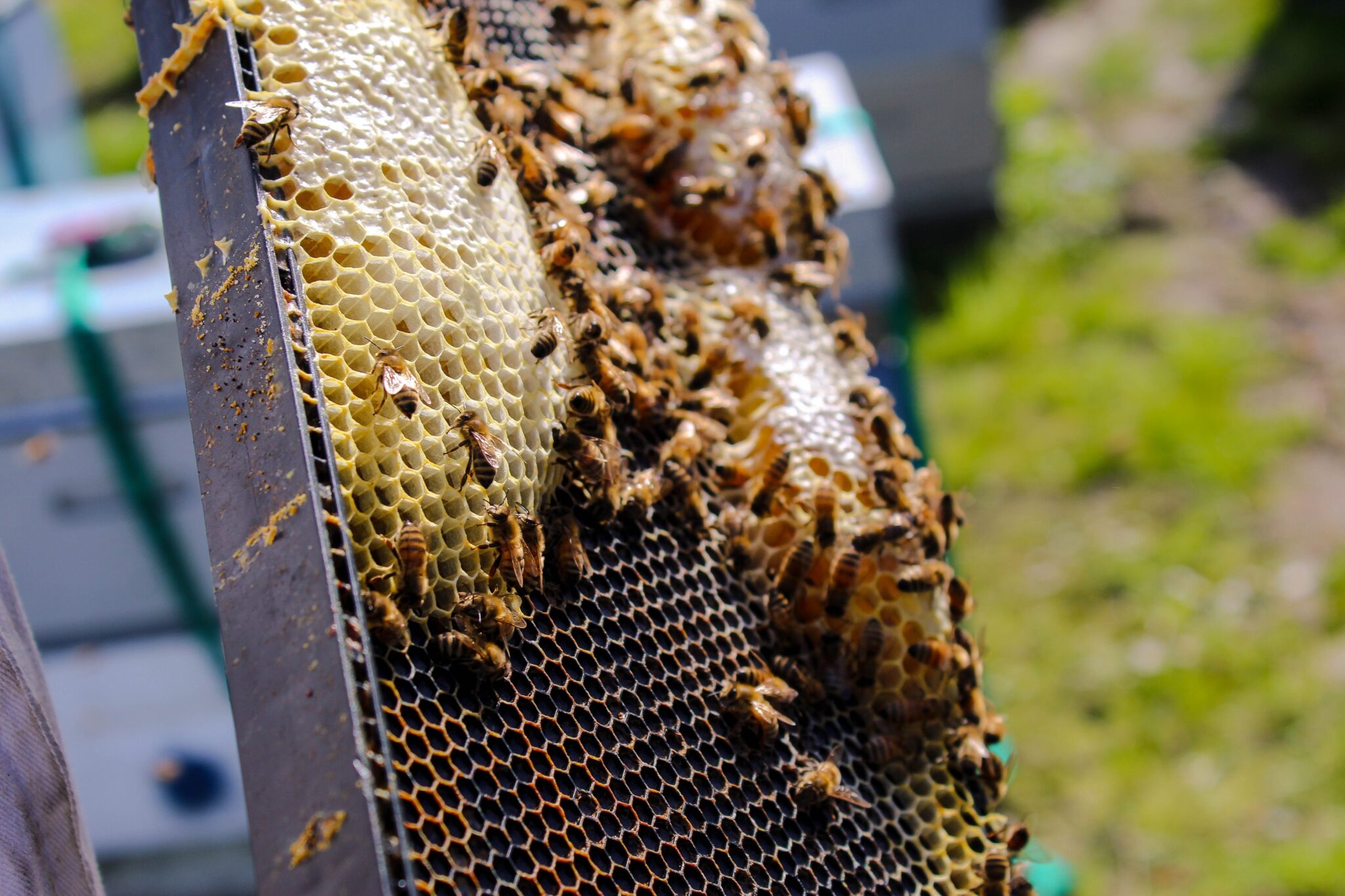
Blog
How to be more bee-like (and go from richer innovation maps to better innovation policies)
15 March 2018
In his Novum Organum, pioneer of the scientific method Francis Bacon compared researchers to animals: there was the ant, which laboriously collected and classified new facts, the spider, which spun theories disconnected from empirical reality, and the bee, which arranged facts in regular structures (theories) that contain new knowledge. According to Bacon, only the bee was doing the true job of philosophy (what we would today call science).
In this blog post I argue that innovation mappers who are using new data to measure innovation have too much of the ant and perhaps not enough of the bee: they are able to measure with increasing detail and timeliness important aspects of the innovation system but if they do not connect their efforts with theories of creativity, innovation, local economic development and growth, all these data will be of limited use for policy. I describe two potential strategies to make these connections happen, and highlight three areas of innovation theory where innovation mapping stands to make valuable contributions.
Innovation mapping today: about to get caught in Borges’ trap?
Where is innovation happening? Who is doing it? What do we do about it?
These are questions for which existing datasets only give us incomplete answers. Official business and innovation surveys structured around standard codes miss new industries such as Fintech, the Internet of Things or Big data. Traditional innovation indicators such as patents exclude innovators in services or creative sectors, and aggregate statistics provide high level national or regional pictures that are not relevant for cluster policies or used to identify individual actors for better policy design and implementation (Bakhshi and Mateos-Garcia, 2016). New datasets, analytics methods and visualisation tools give us the opportunity to address some of these important gaps in the evidence base. Innovation mapping seeks to harness these opportunities, generating timely and granular information which is presented visually to help policymakers navigate complex innovation systems.
Nesta’s is actively involved in this space through projects to map innovation in Wales and the EU, monitor health innovations globally, analyse the immersive technology economy in the UK, and track global AI research trends with pre-print data (Mateos-Garcia and Stathoulopoulos, 2017, Klinger, Mateos-Garcia and Stathoulopoulos, 2018).
Like all acts of measurement, these innovation mapping efforts are implicitly based on a theory: we care about the geography of innovation because following Marshall and Jacobs, we recognise the importance of place in the diffusion of knowledge and the effectiveness of collaboration; we are interested in startups because we have known since Schumpeter that they can innovate more radically than larger incumbents; we analyse the supply of knowledge and skills from universities because as Lundvall told us long ago, innovation happens in systems spanning industry, academia, government and wider society.
So our maps are based on theory. But do they inform it?
Not so much, at least for now. Most innovation mapping efforts so far are like the work of Bacon’s ant, providing descriptions of levels and changes in innovation activities in certain locations and sectors (how many businesses, how many communities, how many research papers?), and ranking innovation ecosystems based on the presence or absence of a variety of local resources and capabilities (who is stronger in a metric or composite of metrics, what’s missing?).
When presenting this work I find that the reaction depends on the audience: while policymakers tend to be positive, some researchers can be quite negative, and not just because of concerns about the quality of data not explicitly collected for research. Some of them question the usefulness of all these data. I believe that while this scepticism reveals some lack of empathy for the needs of policymakers who demand accurate and timely descriptions of the world, I agree with critics that better measuring and mapping of innovation on its own is not enough. In addition to answering the ‘what’, the ‘when’ and the ‘where’, our innovation maps also need to help explain the ‘why’, and predicting ‘what’s next’: they need to inform theory. Innovation mappers need to become more bee-like.
Without this, there is a risk is that the field of innovation mapping ends caught in the trap described by Jorge Luis Borges in his short story ‘On Exactitude in Science’: producing ever more detailed and comprehensive maps of the territory that, for lack of theory, do little to enhance understanding, or are even detrimental to it by generating information overload, a ‘metric tide’, or ‘black box’ findings and predictions which are hard to interpret or explain and therefore less suitable for policy-making.
Paths forward: Observing and experimenting
How do we avoid this trap, and go from richer innovation maps to better innovation theories – and policies? We need to build stronger ties between measurement (mapping phenomena) and hypothesis-testing, which can be based on observation or experimentation.
On observation:
We need to integrate data flowing from many streams about the various resources and capabilities which together contribute to the evolution and performance of innovation systems and clusters. Triangulate these data against ground truths based on traditional datasets and the local knowledge of domain experts. This integration and enrichment would help us to model innovation processes while holding other important factors constant, incorporating it into the analysis interactions between variables, generate artificial control groups (as with propensity score matching methods) and remove noise from the data. Ultimately, this would result in better models of innovation, and more sophisticated tools that present relevant information to policy users and even recommend action based on a more nuanced understanding of the local situation and its causal drivers.
Getting there will require more sophisticated approaches to data integration than dominant practice, which is often simply to lump together many different variables in national, regional or municipal geographies, and then look for correlations in their geography. It is becoming easier to go beyond this: organisation-level matching is increasingly feasible as more collaborative research grant datasets and business registries are opened up. We used this approach to map university-industry collaborations in Creative Nation.
Natural Language Processing methods that classify entities (papers, business, patents) into finely grained categories can also help match activities. This is an approach we have adopted in a recent project to map Innovation in the United Arab Emirates where we classify a variety of innovation activities (including research, business and skills supply and demand) in highly granular tech sectors to gain a holistic view of strengths and gaps in each of them. We will publish our results soon.
On experimentation:
We need to run tests that take us from innovation mapping data to better insights and policies. Experimentation, especially where it is based on rigorous methodologies such as randomised control trials, removes some (if not all) of the need for data integration to control for hard to measure variables, and makes it possible to causally link treatments with outcomes – in other words, to validate policy hypotheses and test theories about innovation and its dynamics.
Innovation mapping can support and enhance this experimentation at different stages of the policy process, from policy design to evaluation. An example of the first case would be to use information about the structure of networks based on social media data to design new brokerage or collaboration interventions that can be monitored using those same data. An example of the second would be using innovation mapping variables to proxy innovation outcomes in a policy intervention. Innovation mapping tools can themselves become experimentation sites, by showing users different types of information and analysing changes in their behaviour and outcomes. This A-B testing model has proven very useful in internet industries and policy areas influenced by behavioural economics and ‘nudging’. Innovation policymakers have much to learn from it. Nesta’s Innovation Growth Lab specialises in the use of these methods and we are actively exploring options to integrate them with innovation mapping data.
Relatedly, good innovation mapping data collected continuously and at a high level of detail can be used to inform theory in quasi-experimental settings where we can follow the repercussions of a ‘shock’ to a system in these data. We are pursuing an opportunity along these lines in a forthcoming paper that analyses the impact of Deep Learning (DL), a new paradigm for Artificial Intelligence (AI) research, on the geography of activity in that domain. We conceptualise the arrival of DL as a shock enabling us to compare the situation in ‘treated’ disciplines where the method has been rapidly adopted with those where it has not (the control). Our findings so far suggest that fields with fast adoption of DL have seen much more volatility in their geography than those that did not, consistent with the idea that disruptive technology shocks can change the innovation fortunes of nations.
Three ways in which innovation mapping can contribute to innovation theory
When used together with analytical methods to develop theory, innovation maps become sensors and measurement devices for a new science of innovation. We know from the history of science that the arrival of new measurement tools has brought with it breakthroughs in theory. Perhaps innovation mapping could do something similar for our understanding of innovation, and the development of better innovation policies. Here are some areas of opportunity:
1. Economic complexity
Innovation mapping and its methods could make a strong contribution to our understanding of economic complexity. This field, pioneered by Ricardo Hausmann at Harvard and César Hidalgo at MIT, conceives an economy as a network of interconnected ‘chunks’ of knowledge that can be recombined, like letters in scrabble, into new products and services. If you have the ‘IT’ letter and the ‘Manufacturing’ letter, then you can combine them into an ‘Internet of Things’ letter. Those economies that are more complex (have more types of knowledge) are better able to innovate, an activity which in itself creates more knowledge to be recombined in a cycle of ever-increasing complexity.
Economic complexity researchers map these networks of knowledge, identify the position of different countries in them, and study how they could travel through the network in a journey of economic development (see figure below for a visualisation of one of those product spaces for the UK – coloured nodes are those where the UK already has a comparative advantage).
Innovation mapping can contribute to this work in a variety of ways, such as, by enriching the set of activities that can be measured when mapping a location’s opportunity space, going beyond exports, patents and scientific publications (the main data sources currently used to measure economic complexity). While enabling analyses at the sub-national level that will be relevant for regional science scholars and cluster policymakers.
The figure below shows a ‘tech space’ we created for Arloesiadur, our innovation mapping project for Welsh Government, which uses data from meetup, a popular events website. We display the connections between different ‘tech topics’ based on their co-occurrence in tech communities in the UK, and identify those topics where Wales specialises. By monitoring how these patterns of specialisation develop we can start gaining an understanding of how an economy can develop capabilities in high-potential services and digital sectors poorly captured in export and patent data.
2. Knowledge spillovers
The economic complexity framework tells us a lot about the ‘knowledge building blocks’ of an economy but not so much about the processes through which they are recombined: do new knowledge combinations happen through imitation, collaboration or movements of people between organisations? What settings – types of businesses, research projects and institutions – are better at creating these combinations? What is the role of ICT platforms whose users combine local knowledge with ‘global pipelines’ of research and innovation activity?
All these factors are important in shaping ‘agglomeration economies’ (the tendency towards geographical concentration of economic activity leading to the emergence of clusters) but we still do not understand very well their relative importance in different scientific disciplines, sectors and industrial structures.
Innovation mapping can help address this: rich datasets about what happens inside individual businesses based on combinations of patent and web data can illuminate patterns of knowledge diffusion which cannot be understood with industrial codes that classify each company in a single sector. Mapping collaboration networks at a rich level of detail can help us understand which types of knowledge ‘travel faster’ and which ones require more face-to-face interaction and flow of people between organisations, potentially informing better networking interventions.
Creative Nation started looking at some of these questions, particularly in its analysis of the embeddedness of creative capabilities in the rest of the economy (the chart below displays how those capabilities -in the vertical axis- are present in other sectors – in the horizontal axis – for the whole of the UK).
When we perform this analysis at the local level, we find that businesses outside of the creative industries (e.g. manufacturing) who are co-located with creative businesses in a sector (e.g. design) tend to display creative capabilities in that same sector (that is, manufacturing companies close to designers tend to talk about design more). We see this kind of correlation as initial evidence for ‘creative knowledge spillovers’ through which creative clustering indirectly benefits the local economy. Innovation mapping methods can feed into the analysis of these spillovers and their mechanisms, strengthening our understanding of how clusters grow and develop.
3. Emergence
Ultimately, recombinations of knowledge result in new technologies, industries and clusters. Policymakers, investors and businesses want to track these processes more precisely and closer to real-time in order to identify the next big thing. But in order to do this we need to leave behind existing industrial, scientific and technology categories which by definition ignore new, emergent areas. We also need to deal with the fact that ‘emerging’ topics are inherently fuzzy and noisy, which can create ‘survivor biases’ in the data (the risk that we only analyse successfully emerged technologies, but not those that could have emerged but failed to).
A new literature has started to develop around this area, providing operational definitions of emerging scientific or technology areas, and analysing the factors driving it. Innovation mapping has a lot to contribute to it: its natural language processing and clustering methods can help to identify emerging topics in large bodies of unstructured text; some of the datasets it uses (such as tech meetup activity, software development or scientific pre-prints) are less laggy than patents and publications and can help to generate a more timely picture of processes of emergence. When it is combined with other datasets, it can help us understand what kinds of networks and ecosystems are better at generating novel ideas.
The aforementioned paper on Deep Learning illustrates some of this. In it, we draw on a very timely scientific pre-prints dataset (ArXiv) where we identify the emergence of a new scientific technique (Deep Learning) by analysing the abstracts of hundreds of thousands of paper using text mining (topic modelling) methods. This makes it possible to not only study how and where this new technique has emerged, but also how it has diffused and become dominant in other computer science disciplines (the chart below illustrates its expansion in fields like Computer Vision, Sound or Language, and the growing importance of such ‘high DL adoption’ fields in computer science). An interesting next step would be to use that same method to identify other topics following a similar pattern of development initially but which were eventually abandoned, and compare their circumstances with Deep Learning.
Making bee-lines from theory to praxis
Connecting mapping and theory is not a purely abstract exercise: it can also yield important policy implications: the three research areas I just mentioned have very significant applications in industrial, cluster and research and innovation policy, addressing key questions about how to build resilient economies, implement smart specialisations, inform daring diversifications and crossover (not least to address ‘innovation missions’) and harness the benefits of emerging technologies for more people.
Advancing our state of knowledge in each of them, and communicating their findings through interactive tools and visualisations that respond to the various needs of policy users and practitioners could generate many practical benefits. Providing better information for the innovation policies of today, and a data springboard for the innovation policies of tomorrow.
Getting there will require more interdisciplinary collaboration, stronger crossovers between data development, research and policy, and a experimental mindset in government, both in terms of the data sources that are used, and how they are integrated in policy programmes to facilitate learning – something akin to the ‘system optimiser role’ we identified in our analysis of how innovation agencies work . None of those are easy challenges, but tackling them could create great benefits.
We couldn’t think of a better place to be.
Get in touch at [email protected] if you are interested in discussing any of this further. We want to talk with policymakers about their needs, questions and applications, with researchers about potential collaborations that use innovation mapping to advance theory, and with fellow mappers about strategies to push the field forward.
Acknowledgements
An initial version of this essay was presented at a workshop on Advanced Mapping Methodologies organised by Alex Kleibrink from the Joint Research Centre (JRC) in Seville in January 2018. It received useful comments from several participants including Gaston Heimeriks, Daniele Rotolo and Jan-Philipp Kramer. The first draft also received valuable feedback from Geoff Mulgan, Kirsten Bound and Albert Bravo Biosca.
The hexagon figure that illustrates the blog was obtained from Wikipedia.
This blog was originally published on the Nesta website on 1 March 2018. You can read the original here.