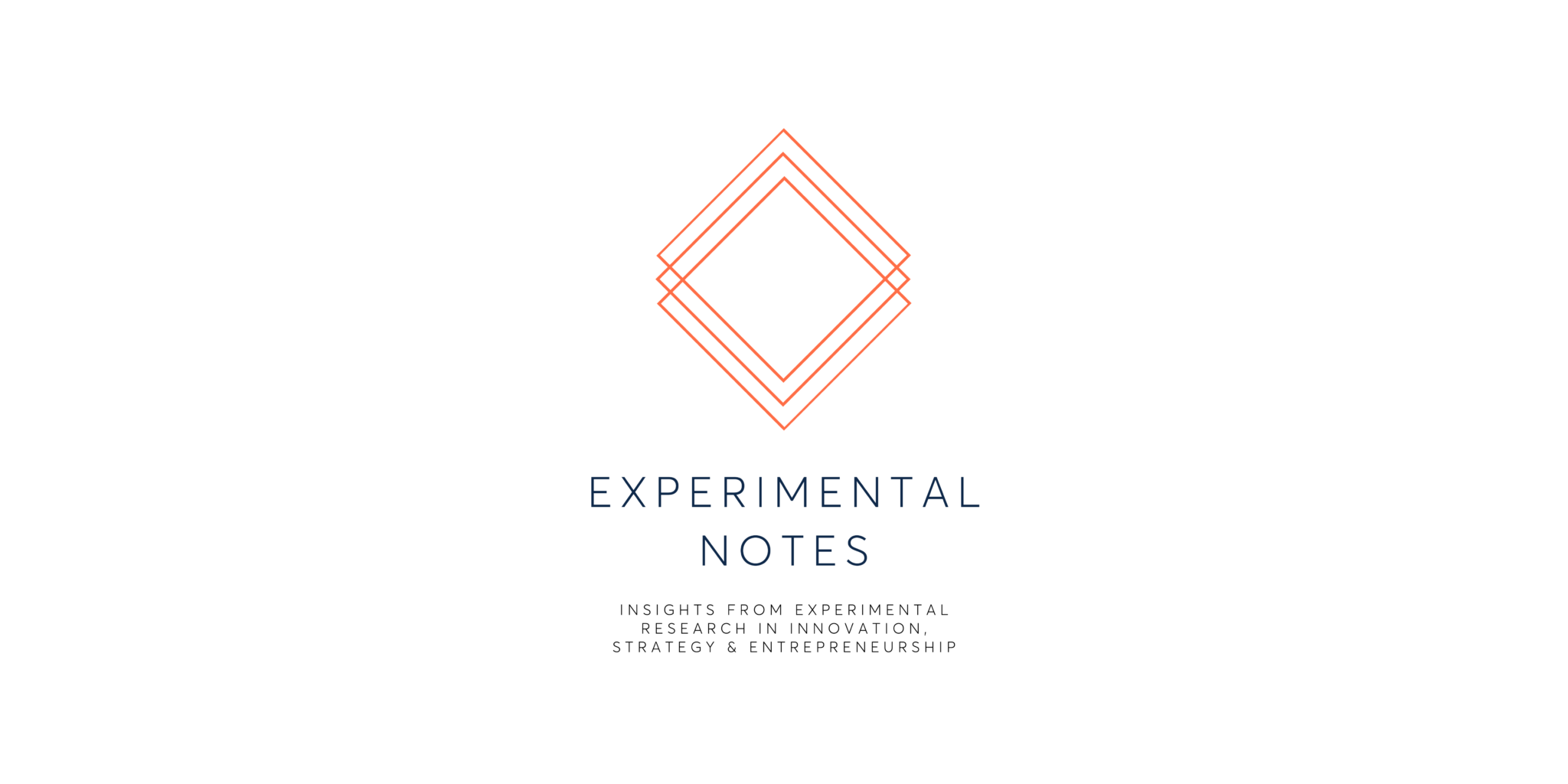
Blog
Experimental Notes: The Second Edition
9 March 2022
Welcome to the second edition of Experimental Notes, a newsletter brought to you by the Workshop on Field Experiments in Strategy, Innovation, and Entrepreneurship and the Innovation Growth Lab. In our last newsletter, we brought you a dozen new papers by scholars interested in questions ranging from the entrepreneurial decision-making process to incentives and team design in innovation contexts. This time, we are still bringing you a dozen new papers, but we are also taking a deeper dive into a key issue that researchers running studies focused on firms are especially likely to face: statistical power.
Before turning to our discussion of power, we want to first remind you that you can forward this newsletter to your friends, students, and colleagues who want to learn more about field experiments focused on innovation, entrepreneurship, and strategy. Also, remind them to sign up for the newsletter themselves. Here is the signup link. Hundreds of researchers have already signed up, but we are keen to keep growing this community. Also, if you have any ideas for newsletter topics (or want to write for us), reach out!
Speaking of community, our Annual Conference will be held on September 15th and 16th in London, UK. Please hold these dates! While the dates are still tentative, we are hoping to hold an in-person conference on that Thursday and Friday, right before the annual Strategic Management Society (SMS) conference, which will also be held in London. We are looking forward to seeing you all there and will be in touch with more details about paper submissions and registration. We will also have a virtual option if travel is not possible for you.
The pursuit of power?
The tradeoff between power and relevance when building your sample
As experimentalists, when we talk about sample size, we almost always start our conversations by focusing on our need for power: statistical power. Power is the likelihood that a significance test detects an effect of the treatment when there is, indeed, an effect. When framed in this way, we always hope for a larger sample. But for most of us, the sample size we want is often not attainable: many studies in our fields have sample sizes in the 100 to 300 range, sometimes much smaller. While our budgets often cap the sample size, the total population is sometimes just as limiting, especially when our unit of analysis is a company or firm. For example, in 2020, just over 600 U.S. startups raised a Series A round for the first time across any industry, be it biology or software as a service! Should researchers focused on high-growth entrepreneurship stop doing field experiments? What should we do when it’s impossible to get a sample size in the thousands, let alone a clustered randomized design?
While Reviewer #2 may disagree, we think researchers should still experiment in the field. Well-designed field experiments across smaller samples can provide important insights. In fact, sometimes the pursuit of power by building a giant sample can undermine an otherwise great research design. Why? Because researchers–especially those studying firms–often face a tradeoff between the size of their sample and the relevance of the sample for their research question. We see this tradeoff as rooted in three underlying issues.
The first issue is a basic one that many of us face regarding how representative the sample is of the population we are interested in studying versus testing on a less representative but larger sample that increases our ability to estimate a treatment effect. Often, researchers start by generating an idea that applies to a specific set of people or firms. For instance, a researcher might be interested in how incentives affect the behavior of managers or how improved judgment might affect the performance of startups. But because of the difficulty of getting enough real startups in their study, they do the study with ‘aspiring entrepreneurs’ or their students. Sometimes this is the right move because we think the underlying mechanism will not vary across different populations. However, there are many instances where we think there will be substantial variation in mechanisms and so treatment effects across different populations. In these cases, choosing the larger but less relevant sample can sacrifice the main value of running a field experiment – to understand the real effects of treatment in practice in an actual population of interest. For instance, showing that peer advice affects an undergraduate in a hackathon with no business experience is not especially informative about the impact of peer advice for a founder with 20 years of experience and 30 employees, who has raised millions of dollars from an experienced VC. In this case, the researcher has to ask whether a study with 100 real entrepreneurs provides more insight about a mechanism, albeit with more statistical uncertainty than a study with 1,000 undergraduates. While there may not be a single correct answer, researchers should be upfront about the trade-offs they have made in choosing a small but relevant sample over a large but potentially less relevant one.
Indeed, some fantastic papers show the value of going for a smaller sample but one with high relevance. The well-known paper “Does Management Matter? Evidence from India” by Bloom et al. (2013) has 17 firms, with 11 in the treatment group and 6 in the control group. That number rises to only 14 and 6 when counting individual plants, rather than firms, as the relevant unit. However, the choice to use these firms and a smaller sample are incredibly well-motivated. The research question is focused on management practices in large and real firms — specifically those that have a large number of employees (270 in their case), substantial assets ($13 million), and considerable sales ($7.5 million). Indeed, the firms they study are among the top 1% of firms in India in employment and sales and would rank among the top 2% to 4% even in the United States. Another great example is Atkin et al. (2017), “Exporting and Firm Performance: Evidence from a Randomized Experiment,” which examines the role of exporting on learning and firm performance among small-scale rug manufacturers in Pakistan. The total population of firms they could study was 303, with only a tiny fraction (10% or 32) willing to be treated. Indeed, Atkin et al. note that “Even among the 79 specialist duble [a type of rug] producers, only 14 of the 39 treated firms took up, with nontakeup firms citing an unwillingness to jeopardize their existing dealer relationships for the small 10–20 m2 order sizes.” This rich detail provides greater context into how much their treatment effect can be generalized and to whom. Returning to startup advice, in “When does advice impact startup performance?” by Chatterji et al. (2019) uses a peer effects field experiment with only 100 high-growth startups to show better management advice improves startup growth. However, the paper also reveals why testing on a sample of undergraduates would have been less informative. The authors show this advice only matters when founders hadn’t already received formal training as part of an MBA or accelerator. This variation helps us understand when advice will matter for startup performance.
If you have other examples of small but highly-relevant samples in studies to share, either from your papers or those that you have read – contact us at [email protected]. We’d love to hear from you and share them with the rest of the community.
The second relevance-size tradeoff stems from the problem of participant-driven selection into the experiment that Atkin et al. (2017) discuss. Their scenario shows that those willing to participate in their experiment don’t have the same stress about jeopardizing relationships with existing buyers. Perhaps, the reduced stress (and potential for internal tradeoffs) for those that select into treatment makes it easier for them to implement exporting and learn from it – thereby delivering a larger treatment effect than would be possible in a broader pool of firms. This issue is a version of the “Site selection bias” problem raised by Allcott (2015), who shows that the unobserved willingness of sites to be part of an experiment leads to an overestimation of the population-level treatment effect. This bias may sometimes lead to underestimating effects, especially when studying firms and entrepreneurs whose opportunity costs of participation can be quite high.
This selection problem means that ideally, field experiments should be run across the entire population when possible. This can sometimes be more feasible when collaborating with the government or with online platforms, such as in “Do Labor Market Policies Have Displacement Effects?” by Crepon et al (2013) on job placement assistance in France and “The Value of Competitor Information” by Kim (2021) on how firms change their strategy in response to competitor information in the personal care industry across key US markets. In some instances, doing so may also allow us to evaluate heterogeneity in treatment take-up and site selection bias. Of course, even in these cases, selection bias needs to be taken into account when generalizing to a higher-level population like firms across other markets or countries.
But, anyone who has ever conducted a field experiment knows how hard it is to recruit subjects – especially firms. Unlike researchers focused on individual-level behavior who can often turn to professionally managed subject pools like Prolific, getting high-skilled workers or firms to participate in a study is incredibly difficult. We are often lucky to get anyone to participate, let alone the relevant people or firms. This challenge is reasonable. Anyone with a growing business or busy job is likely to think twice before committing time and effort to become a participant in a research project. Consequently, we may be getting some degree of unintended and unobservable selection into our experiments — although of course, this problem does not only exist for field experiments. Any empirical analysis has to choose a sample from a specific setting, and firms that collect high-quality data necessary for observational analyses may also have systematically different attributes that can generate biased estimates.
When running an experiment across the entire population of interest is not possible, a smaller, well-designed sample can often provide more informative estimates than a larger one without design considerations. For example, rather than having a sample of all firms that select in, a smaller number of firms can be randomly selected within a strata of observable attributes that are potentially relevant. In a similar vein, researchers can focus on a more specific, smaller population of interest to target and spend more effort and resources to recruit them. In fact, a recent working paper by Crama et al. “Which Businesses Enroll in Innovation Training? Evidence from a Field Experiment” uses 10,000 firms targeted for an experiment to study potentially biased selection into enrollment. They find that only a tiny handful of targeted firms enroll (about 2%), but the most striking is that the enrolling firms are substantially smaller and doing quite poorly in recent years. If these individuals come from businesses that are doomed to fail, a ‘large scale’ recruitment strategy may also doom the treatment effect to be zero. Conversely, if a researcher spends more effort (and money) vetting and recruiting each subject, they may well end up with a much smaller sample, but one where their treatment has at least a fair chance of working. The take-away: A smaller, but more relevant sample that you can characterize viz-a-viz the larger population, may be more likely to give your treatment a fair shot and give you a sense of where your findings may or may not apply.
The third issue related to the relevance-size tradeoff relates to how we should think about designing our intervention given our sample, especially when it is on the smaller side. Most researchers have two far from perfectly aligned goals: (a) uncovering a specific mechanism and (b) quantifying the effect of a treatment. While there is much emphasis on uncovering mechanisms in order to make theoretical contributions in the social sciences, the unfortunate truth is that a specific mechanism may have small effects in isolation and be hard to tease apart (especially in small samples). In addition, it may in fact be impossible in many cases for a firm to practically implement a single mechanism alone. As such, the researcher may need to bundle lots of mechanisms together to ensure that the effect is large enough to detect in relatively modest samples. The Bloom et al. study of textile firms provides an excellent section on their bundled treatment, including five items ranging from factor operations to sales and order management (see III.B Management Consulting Intervention).
One helpful approach to thinking about how to “bundle” mechanisms together is to think of them as belonging to ‘classes’ or ‘types’ rather than operating in some independent or atomistic way. One way to do this is to find interventions that are often implemented together in practice — in other words, to evaluate a practically relevant policy or program, rather than designing a mechanism experiment (borrowing the language of Ludwig et al 2011). The Bloom et al. study is a good example of this, as it looks at the impact of receiving consulting on management practices, leveraging a globally leading consultancy that many firms engage. Similarly, recent work by Dimitriadis and Koning (2021) evaluates how “bundled” social skills training impacts small business performance in a sample of just under 300 entrepreneurs. To maximize the treatment effects, the authors designed a multi-faceted “social skills” training module loosely modeled on a popular course offered to MBAs at Stanford GSB. On the one hand, this “bundled treatment” prevents them from teasing apart whether any individual mechanism like better networking, improved communication, or shifts in willingness to collaborate is definitely at play. On the other, by moving many mechanisms the authors improved the chances their treatment would have an effect, perhaps some entrepreneurs benefited from the networking and others from how to talk about their business. Returning to the relevance-size tradeoff, when you have a limited sample size to work with, make sure your treatment is relevant on multiple dimensions to ensure that the effect is large enough for you to detect.
If you have other examples of theoretically tight treatment bundles in studies to share, either from your papers or those that you have read – we’d love to share them with the rest of the community.
Lastly, given this relevance-size tradeoff, maybe it’s worth re-examining how we think about what the “minimum viable experiment” is for a research paper. For many of us, a field experiment is often an event. It is expensive, time-consuming, and high-stakes. If it fails, there go years of work, and we might not learn anything at all, let alone get it published. Instead, we might consider structuring experimentation in our world into “phases” like in drug trials that embrace experimentation in a broader sense. In drug RCTs, a phase 1 trial may be a small sample study with 50-100 subjects trying out a new treatment. If the phase 1 experiment works, a more extensive study may be warranted to estimate the treatment effect better. If phase 2 goes well, an even more extensive study might be conducted to understand mechanisms, heterogeneity, and other nuances that could help eventual implementation and rollout. Each phase gets written up and published. This model reduces the stakes for the first experiment. In doing so, it helps researchers both accumulate more robust evidence and creates incentives to get more creative, trying out new ideas that would simply be too risky in higher-stakes, big N, trials. By reconsidering our need for larger samples perhaps we can also think more experimentally about our experiments.
What we’re reading
Algorithms and Firm Decisions
AI Training for Online Entrepreneurs: An Experiment with Two Million New Sellers on an E-commerce Platform
Yizhou Jin & Zhengyun Sun
We collaborate with a large e-commerce platform and conduct a year-long experiment among new sellers on the platform. The treatment group receives access to a free and customized entrepreneur training program, in which an AI algorithm dynamically assigns training materials to sellers based on their operations data. With a 24% take-up rate, new sellers that are eligible for training see 1.7% higher revenue on average (6.6% ATT), largely driven by higher traffic and enhanced marketing activities. To investigate consumer-side benefit while boosting statistical power, we construct a panel dataset of consumer-seller pairs across consideration sets that are observed in search sessions. Using exhaustive controls and fixed effects, we show that training also improves new-seller conversion, which is not driven by selection through heightened entry barriers but due to a direct effect on quality. We then estimate an empirical model to capture unobserved consumer preference heterogeneity and compute welfare. Although only 0.25% of products in consumers’ consideration sets are from treated new sellers, removing the program would reduce consumer surplus by 0.07%.
Decision Authority and the Returns to Algorithms
Edward L. Glaeser, Andrew Hillis, Hyunjin Kim, Scott Duke Kominers, & Michael Luca
We evaluate a pilot in an Inspections Department to test the returns to a pair of algorithms that varied in their sophistication. We find that both algorithms provided substantial prediction gains over inspectors, suggesting that even simple data may be helpful. However, these gains did not result in improved decisions. Inspectors used their decision authority to override algorithmic recommendations, without improving decisions based on other organizational objectives. Interviews with 29 departments find that while many ran similar pilots, all provided considerable decision authority to inspectors, and those with sophisticated pilots transitioned to simpler approaches. These findings suggest that for algorithms to improve managerial decisions, organizations must consider the returns to algorithmic sophistication in each context, and carefully manage how decision authority is allocated and used.
Testing New Ideas
A Scientific Approach to Innovation Management: Theory and Evidence from Four Field Experiments
Arnaldo Camuffo, Alfonso Gambardella, Danilo Messinese, Elena Novelli, Emilio Paolucci & Chiara Spina
This paper studies the implications of an approach in which managers and entrepreneurs make decisions under uncertainty by formulating and testing theories such as scientists do. By combining the results of four Randomized Control Trials (RCTs) involving 754 start-ups and small-medium enterprises and 10,730 data points over time, we find that managers and entrepreneurs who adopt this approach terminate more projects, do not experiment with many new ideas, and perform better. We develop a model that explains these results.
The Signup Problem
Promoting Platform Takeoff and Self-Fulfilling Expectations: Field Experimental Evidence
Kevin Boudreau
A platform might have the potential to bring enormous value to its users. However, without a well-orchestrated launch strategy that coordinates a sufficient number of users onto the platform, this potential will not be realized. The theoretical literature predicts that one approach to coordinating platform take-off is to influence the market’s subjective focal expectations of the future installed base of users.This paper reports on a field experiment investigating the causal role of subjective expectations in the launch of a new platform venture, in which invitations to join a newly launched platform were sent to 16,349 individuals. The invitations included randomized statements regarding the size of the future expected installed base (along with disclosures of the current installed base). I find that simple, subjective, uncommitted, and relatively costless statements broadcasted by the platform with the goal of influencing market expectations were indeed able to influence platform takeoff and overcome an initial chicken-and-egg problem. These broadcasted subjective statements regarding future installed base had a larger influence on adoption rates than did disclosures of the true current installed base during early adoption. However, these subjective statements of expected future installed base ceased to have any effect once the true current installed base grew large. I discuss implications for the promotion, marketing, and evangelism of new platform ventures.
What Motivates Innovative Entrepreneurs? Evidence from a Global Field Experiment
Jorge Guzman, Jean Joohyun Oh & Ananya Sen
Entrepreneurial motivation is important to the process of economic growth. However, evidence on the motivations of innovative entrepreneurs, and how those motivations differ across fundamental characteristics, remains scant. We conduct three interrelated field experiments with the Massachusetts Institute of Technology Inclusive Innovation Challenge to study how innovative entrepreneurs respond to messages of money and social impact and how this varies across gender and culture. We find consistent evidence that women and individuals located in more altruistic cultures are more motivated by social-impact messages than money, whereas men and those in less altruistic cultures are more motivated by money than social impact. The estimates are not driven by differences in the type of company, its size, or other observable characteristics, but, instead, appear to come from differences in the underlying motivations of innovative entrepreneurs themselves.
Which Businesses Enroll in Innovation Training? Evidence from a Field Experiment
Pascale Crama, Sharique Hasan, Reddi Kotha, Vish Krishnan, Cintia Sacilotto Kulzer, Cintia and Lim
We report results from a field experiment testing hypotheses that examine what drives firms to seek new learning opportunities. Specifically, we draw on behavioral theory of the firm to predict how prior performance affects the likelihood a firm enrolls in business training. We also evaluate cognitive mechanisms connecting recruitment messaging and CEO growth orientation to firm participation. Our study randomly allocates over 10,000 firms to one of three experimental conditions—prevention, promotion, and neutral messaging—that vary the framing of a recruitment message for an innovation program for small and medium enterprises (SMEs) in Singapore. We leverage pre-treatment heterogeneity in firm performance and CEO orientation to better understand the differential impact of the three message types. We find that businesses with declining performance are 64% more likely to register than those with performance improving year over year. In addition, we find mixed evidence of a congruence effect—where messages (i.e., promotion) resonate more with CEOs with matching orientations. Surprisingly, we find that the neutral messaging performs 46% better than the promotion message and 115% better than the prevention message in spurring enrollment. Our work sheds light on both the frictions and remedies for scaling up the diffusion of new knowledge to businesses. Specifically, we find that subtle differences in recruitment strategy affect who enrolls and the overall demand for business training. Overall, our findings suggest that targeted firm performance-heterogeneity and the varied experimental recruitment efforts significantly affect enrolment. Researchers must pay careful attention to selection in attempting to understand who benefits from the training.
Evaluation Design
The Risk of Caution: Evidence from an Experiment
Richard T. Carson, Joshua Graff Zivin, Jordan J. Louviere, Sally Sadoff & Jeffrey G. Shrader
Innovation is important for firm performance and broader economic growth. However, breakthrough innovations necessarily require greater risk taking than more incremental approaches. To understand how managers respond to uncertainty when making research and development decisions, we conducted experiments with master’s degree students in a program focused on the intersection of business and technology. Study participants were asked to choose whether to fund hypothetical research projects using a process that mirrors real-world research and development funding decisions. The experiments provided financial rewards that disproportionately encouraged the choice of higher-risk projects. Despite these incentives, most participants chose lower-risk projects at the expense of projects more likely to generate a large payoff. Heterogeneity analysis and additional experimental treatments show that individual risk preferences predict greater tolerance of high-risk projects and suggest that more appropriate decision making can be learned. Thus, for firms seeking to fund breakthrough research and development, appropriate screening and training of employees may play important roles in increasing the likelihood of success.
Social Networks: Online and Offline
Virtual Watercoolers: A Field Experiment on Virtual Synchronous Interactions and Performance of Organizational Newcomers
Iavor Bojinov, Iavor and Choudhury & Jacqueline N. Lane
Do virtual, yet informal and synchronous, interactions affect individual performance outcomes of organizational newcomers? We report results from a randomized field experiment conducted at a large global organization that estimates the performance effects of ‘virtual water coolers’ for remote interns participating in the firm’s flagship summer internship program. Findings indicate that interns who had randomized opportunities to interact synchronously and informally with senior managers were significantly more likely to receive offers for full-time employment, achieved higher weekly performance ratings, and had more positive attitudes toward their remote internships. Further, we observed stronger results when the interns and senior managers were demographically similar. Secondary results also hint at a possible abductive explanation of the performance effects: virtual watercoolers between interns and senior managers may have facilitated knowledge and advice sharing. This study demonstrates that hosting brief virtual water cooler sessions with senior managers might have job and career benefits for organizational newcomers working in remote workplaces, an insight with immediate managerial relevance.
A foot in the door: Field experiments on entrepreneurs’ network activation strategies for investor referrals
Jared Nai, Yimin Lin, Reddi Kotha & Balagopal Vissa
We investigate entrepreneurial network activation—the processes by which entrepreneurs select specific contacts from their existing personal network and persuade the selected contacts to provide referrals to access targeted early-stage investors (venture capitalists or angel-investors). We differentiate between selection of entrepreneur-centric contacts versus investor-centric contacts. We also distinguish between persuasion tactics that induce contacts’ cooperation through promises of reciprocity versus offers of monetary incentives. We conducted two field-experiments in India and one in Singapore. Our primary field-experiment involved 42 Singapore-based entrepreneurs seeking referrals from 684 network contacts to reach a panel of four investors. Our evidence suggests that selecting investor-centric contacts leads to greater referral success; in addition, persuasion by promising reciprocity also leads to greater referral success.
Labor
Salary History and Employer Demand: Evidence from a Two-Sided Audit
Amanda Y. Agan, Bo Cowgill & Laura K. Gee
We study how salary history disclosures affect employer demand by using a novel, two-sided field experiment featuring hundreds of recruiters reviewing over 2000 job applications. We randomize the presence of salary history questions as well as candidates’ disclosures. We find that employers make negative inferences about non-disclosing candidates, and view salary history as a stronger signal about competing options than worker quality. Disclosures by men (and other highly-paid candidates) yield higher salary offers, however they are negative signals of value (net of salary), and thus yield fewer callbacks. Male wage premiums are regarded as a weaker signal of quality than other sources (such as the premiums from working at higher paying firms, or being well-paid compared to peers). Recruiters correctly anticipate that women are less likely to disclose salary history at any level, and punish women less than men for silence. In our simulation of bans, we find no evidence that bans affect the gender ratio of callback choices, but find large reductions in gender inequality in salary offers among candidates called back. However, salary offers are lower overall (especially for men). A theoretical framework shows how these effects may differ by key properties of labor markets.
Middle Managers, Personnel Turnover, and Performance: A Long Term Field Experiment in a Retail Chain
Guido Friebel, Matthias Heinz, & Nikolay Zubanov
In a randomized controlled trial, a large retail chain’s Chief Executive Officer (CEO) sets new goals for the managers of the treated stores by asking them to “do what they can” to reduce the employee quit rate. The treatment decreases the quit rate by a fifth to a quarter, lasting nine months before petering out, but reappearing after a reminder. There is no treatment effect on sales. Further analysis reveals that treated store managers spend more time on human resources (HR) and less on customer service. Our findings show that middle managers are instrumental in reducing personnel turnover, but they face a trade-off between investing in different activities in a multitasking environment with limited resources. The treatment does produce efficiency gains. However, these occur only at the firm level.
Methodology and Theory
Experimenting in Equilibrium
Stefan Wager & Kuang Xu
Classical approaches to experimental design assume that intervening on one unit does not affect other units. There are many important settings, however, where this noninterference assumption does not hold, as when running experiments on supply-side incentives on a ride-sharing platform or subsidies in an energy marketplace. In this paper, we introduce a new approach to experimental design in large-scale stochastic systems with considerable cross-unit interference, under an assumption that the interference is structured enough that it can be captured via mean-field modeling. Our approach enables us to accurately estimate the effect of small changes to system parameters by combining unobtrusive randomization with lightweight modeling, all while remaining in equilibrium. We can then use these estimates to optimize the system by gradient descent. Concretely, we focus on the problem of a platform that seeks to optimize supply-side payments p in a centralized marketplace where different suppliers interact via their effects on the overall supply-demand equilibrium, and we show that our approach enables the platform to optimize p in large systems using vanishingly small perturbations.
Using Clinical Trial Data to Estimate the Costs of Behavioral Interventions for Potential Adopters: A Guide for Trialist
LB Russell, LA Norton & D Pagnotti, et al.
Behavioral interventions involving electronic devices, financial incentives, gamification, and specially trained staff to encourage healthy behaviors are becoming increasingly prevalent and important in health innovation and improvement efforts. Although considerations of cost are key to their wider adoption, cost information is lacking because the resources required cannot be costed using standard administrative billing data. Pragmatic clinical trials that test behavioral interventions are potentially the best and often only source of cost information but rarely incorporate costing studies. This article provides a guide for researchers to help them collect and analyze, during the trial and with little additional effort, the information needed to inform potential adopters of the costs of adopting a behavioral intervention. A key challenge in using trial data is the separation of implementation costs, the costs an adopter would incur, from research costs. Based on experience with 3 randomized clinical trials of behavioral interventions, this article explains how to frame the costing problem, including how to think about costs associated with the control group, and describes methods for collecting data on individual costs: specifications for costing a technology platform that supports the specialized functions required, how to set up a time log to collect data on the time staff spend on implementation, and issues in getting data on device, overhead, and financial incentive costs.
Experimental Design in Two-Sided Platforms: An Analysis of Bias
Ramesh Johari, Hannah Li, Inessa Liskovich, Gabriel Y. Weintraub
We develop an analytical framework to study experimental design in two-sided marketplaces. Many of these experiments exhibit interference, where an intervention applied to one market participant influences the behavior of another participant. This interference leads to biased estimates of the treatment effect of the intervention. We develop a stochastic market model and associated mean field limit to capture dynamics in such experiments and use our model to investigate how the performance of different designs and estimators is affected by marketplace interference effects. Platforms typically use two common experimental designs: demand-side “customer” randomization (CR) and supply- side “listing” randomization (LR), along with their associated estimators. We show that good experimental design depends on market balance; in highly demand-constrained markets, CR is unbiased, whereas LR is biased; conversely, in highly supply-constrained markets, LR is unbiased, whereas CR is biased. We also introduce and study a novel experimental design based on two-sided randomization (TSR) where both customers and listings are randomized to treatment and control. We show that appropriate choices of TSR designs can be unbiased in both extremes of market balance while yielding relatively low bias in intermediate regimes of market balance.
Relational incentives theory
Gallus, Jana Reiff Gallus, Joseph Kamenica, Emir Fiske, & Alan Page
Our life is built around coordinating efforts with others. This usually involves incentivizing others to do things and sustaining our relationship with them. Using the wrong incentives backfires: it lowers effort and tarnishes our relationships. But what constitutes a “wrong” incentive? And can incentives be used to shape relationships in a desired manner? To address these and other questions, we introduce relational incentives theory, which distinguishes between two aspects of incentives: schemes (how the incentive is used) and means (what is used as an incentive). Prior research has focused on means (e.g., monetary vs. nonmonetary incentives). Our theory highlights the importance of schemes, with a focus on how they interact with social relationships. It posits that the efficacy of incentives depends largely on whether the scheme fits the relational structure of the persons involved in the activity: participation incentive schemes for communal sharing relations, hierarchy for authority ranking relations, balancing for equality matching relations, and proportional incentive schemes for market pricing relations. We show that these four schemes encompass some of the most prevalent variants of incentives. We then discuss the antecedents and consequences of the use of congruent and incongruent incentive schemes. We argue that congruent incentives can reinforce the relationship. Incongruent incentives disrupt relational motives, which undermines the coordinating relationship and reduces effort. But, importantly, incongruent incentives can also be used intentionally to shift to a new relational model. The theory thus contributes to research on relational models by showing how people constitute and modulate relationships. It adds to the incentives and contracting literatures by offering a framework for analyzing the structural congruence between incentives and relationships, yielding predictions about the effects of incentives across different organizational and individual-level contexts.
Sign up here to receive Experimental Notes, a quarterly newsletter bringing you fresh research insights from experiments in innovation, strategy and entrepreneurship.